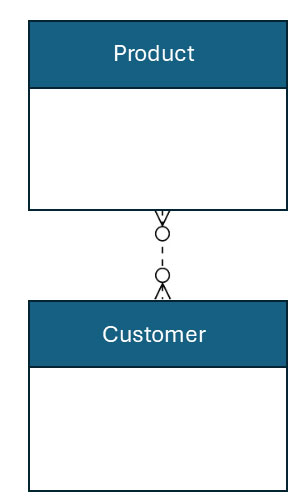
In today’s data-driven world, businesses are inundated with vast amounts of information from various sources. To harness this data effectively, it’s crucial to have a structured approach to organizing and analyzing it. This is where data modeling comes into play. Data modeling creates a visual representation of data structures and relationships within an organization. It is a blueprint that guides how data is stored, accessed, and utilized, ultimately leading to more informed decision-making and effective marketing strategies.
What is Data Modeling?
Data modeling involves the creation of diagrams, schemas, or maps that depict the flow and organization of data within a system. It provides a structured framework for how data elements relate to each other, enabling organizations to understand and manage their data more efficiently. Data models are typically classified into three types:
Conceptual Data Models: These models provide a high-level view of the business concepts and entities that are important to the organization. They are often used in the early stages of a project to define the scope and structure of the data without getting into technical details.
Logical Data Models: Logical data models go a step further by detailing the attributes, relationships, and rules that govern the data. These models are more detailed than conceptual models and serve as a bridge between the business requirements and the technical implementation.
Physical Data Models: Physical data models focus on how data will be stored in a database, including the specific tables, columns, indexes, and constraints. Database developers use these models to implement the data structure in a database management system (DBMS).
Why is Data Modeling Important?
Improved Data Quality: Data modeling ensures that data is organized logically and consistently, which helps to reduce redundancy and errors. By defining clear relationships and rules, data modeling helps maintain data integrity and accuracy across the organization.
Enhanced Decision-Making: A well-structured data model provides a clear view of the available data and its interconnections, making it easier for marketers and analysts to extract meaningful insights. These insights are critical for making informed decisions that drive marketing strategies and business growth.
Streamlined Data Management: Data modeling simplifies the management of data by providing a clear framework for how data is stored and accessed. This structured approach makes it easier to manage large volumes of data, perform complex queries, and maintain data consistency over time.
Effective Collaboration: Data models serve as a common language between business stakeholders, data analysts, and technical teams. By providing a visual representation of data structures, data modeling facilitates better communication and collaboration across departments, ensuring that everyone is aligned on data usage and objectives.
Scalability and Flexibility: As businesses grow, their data needs evolve. Data modeling allows for scalability by providing a framework that can be easily modified to accommodate new data sources, structures, and relationships. This flexibility is crucial for adapting to changing business environments and emerging technologies.
Efficient Data Integration: In today’s multi-platform marketing landscape, data often comes from diverse sources such as CRM systems, social media platforms, email marketing tools, and web analytics. Data modeling helps integrate these disparate data sources into a cohesive structure, enabling seamless data flow and unified analysis.
How Data Modeling Supports Marketing Strategies
Customer Segmentation: Data modeling allows marketers to define and organize customer attributes such as demographics, behavior, purchase history, and preferences. This structured data can then be used to create detailed customer segments, enabling more targeted and personalized marketing campaigns.
Predictive Analytics: By organizing historical data into a coherent structure, data modeling facilitates the application of predictive analytics. Marketers can use these models to identify patterns, forecast trends, and predict customer behavior, allowing for proactive marketing strategies.
Campaign Performance Analysis: Data models help track and analyze key performance indicators (KPIs) across different marketing channels. By structuring data from various campaigns in a unified model, marketers can gain a comprehensive view of what’s working and what’s not, leading to more effective optimization efforts.
Lead Scoring and Nurturing: Data modeling supports the creation of lead-scoring models that rank prospects based on their likelihood to convert. By integrating data from various touchpoints, such as website visits, email interactions, and social media engagement, data models help marketers prioritize leads and tailor nurturing strategies accordingly.
ROI Measurement: With a well-defined data model, marketers can accurately track the return on investment (ROI) for their campaigns. By linking revenue data to specific marketing activities, data models provide a clear picture of how each campaign contributes to the bottom line, enabling better budget allocation and strategy refinement.
Best Practices for Data Modeling in Marketing
Understand Business Requirementscreating: Before creating a data model, it’s essential to understand the business goals and objectives. This ensures that the model aligns with the organization’s needs and supports its marketing strategies.
Start with a Conceptual Model: Begin the data modeling process with a high-level conceptual model that captures the key entities and relationships. This model serves as a foundation for more detailed logical and physical models.
Involve Stakeholders: Throughout the data modeling process, engage key stakeholders from both business and technical teams. Their input is crucial for ensuring the model meets business requirements and is technically feasible.
Document and Communicate: Document the data model and communicate it to all relevant parties. This documentation references future development, maintenance, and data governance efforts.
Regularly Review and Update: Data models should be reviewed and updated to reflect changes in business processes, data sources, and technology. This ongoing maintenance ensures that the model remains relevant and effective.
A Critical Tool for Success
Data modeling is a fundamental aspect of modern marketing that enables organizations to organize, manage, and utilize their data effectively. By creating structured representations of data relationships, businesses can improve data quality, enhance decision-making, and develop more effective marketing strategies. As marketing continues to evolve, data modeling will remain a critical tool for turning raw data into actionable insights, driving business success in an increasingly data-driven world.